On sexual harassment in the workplace (GS Paper 1, Indian Society)
Context:
How was the PoSH Act formed?
- In 1992, Bhanwari Devi, a social worker with the Women’s Development Project of the Rajasthan government was gang-raped by five men after she tried to prevent the marriage of a one-year-old girl.
- While hearing pleas filed by activist groups against the crime, the SC, noting the absence of any law “enacted to provide for effective enforcement of the basic human right of gender equality” guarantee against “sexual harassment at workplaces”, laid down a set of guidelines in 1997, christened the Vishakha Guidelines, to fill the statutory vacuum till a law could be enacted. These were to be “strictly observed in all workplaces” and were binding and enforceable in law.
- After this, the Protection of Women against Sexual Harassment at Workplace Bill was introduced by then Women and Child Development Minister, Krishna Tirath, in 2007. It was later tabled in Parliament and went through amendments.
- The amended Bill came into force on December 9, 2013, as the Sexual Harassment of Women at Workplace (Prevention, Prohibition and Redressal) or PoSh Act.
What is the PoSh Act?
- The PoSH Act defines sexual harassment to include unwelcome acts such as physical contact and sexual advances, a demand or request for sexual favours, making sexually coloured remarks, showing pornography, and any other unwelcome physical, verbal or non-verbal conduct of a sexual nature.
- Under the Act, an employee is defined not just in accordance with the company law. All women employees, whether employed regularly, temporarily, contractually, on an ad hoc or daily wage basis, as apprentices or interns or even employed without the knowledge of the principal employer, can seek redressal to sexual harassment in the workplace.
- The law expands the definition of ‘workplace’ beyond traditional offices to include all kinds of organisations across sectors, even non-traditional workplaces. It applies to all public and private sector organisations throughout India.
What are the requirements imposed on employers?
What are the hurdles to the Act’s implementation?
- The Supreme Court in its recent judgment called out the lacunae in the constitution of ICCs, citing a newspaper report that 16 out of the 30 national sports federations in the country had not constituted an ICC to date.
- The judgment also flagged the improper constitution in cases where the ICCs were established, pointing out that they either had an inadequate number of members or lacked a mandatory external member. This, however, is not the only implementation-related concern when it comes to the PoSH Act.
- One of the concerns is that the Act does not satisfactorily address accountability, not specifying who is in charge of ensuring that workplaces comply with the Act, and who can be held responsible if its provisions are not followed.
- Stakeholders also point out how the law is largely inaccessible to women workers in the informal sector. Additionally, experts have noted that in workplaces sexual harassment cases are hugely underreported for a number of reasons.
- The inefficient functioning and the lack of clarity in the law about how to conduct such inquiries have ended up duplicating the access barriers associated with the justice system.
- The power dynamics of organisations and fear of professional repercussions also stand in the way of women for filing complaints.
What are the SC’s recent directions?
- The court directed the Union, States and UTs to undertake a time-bound exercise to verify whether Ministries, Departments, government organisations, authorities, public sector undertakings, institutions, bodies, etc. had constituted Internal Complaints Committees (ICCs), Local Committees (LCs) and Internal Committees (ICs) under the Act.
- These bodies have been ordered to publish the details of their respective committees in their websites.
What are the gaps in the AePS transaction model?
(GS Paper 3, Science and Technology)
Why in news?
- Cybercriminals are now using silicone thumbs to operate biometric POS devices and biometric ATMs to drain users’ bank accounts.
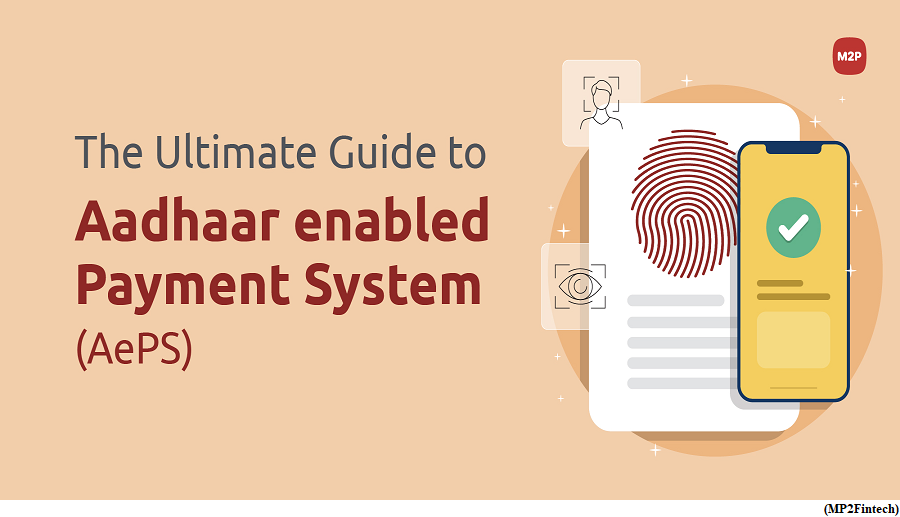
What is AePS?
Is AePs enabled by default?
- Neither the Unique Identification Authority of India (UIDAI) nor NPCI mentions clearly whether AePS is enabled by default.
- The service does not require any activation, with the only requirement being that the user’s bank account should be linked with their Aadhaar number.
- Users who wish to receive any benefit or subsidy under schemes notified under section 7 of the Aadhaar Act, have to mandatorily submit their Aadhaar number to the banking service provider.
How is biometric information leaked?
- While Aadhaar data breaches have been reported in 2018, 2019, and 2022, the UIDAI has denied any breach of data. However, UIDAI’s database is not the only source from where data can be leaked.
- Aadhaar numbers are readily available in the form of photocopies, and soft copies, and criminals are using Aadhaar-enabled payment systems to breach user information.
- Scammers have, in the past, made use of silicone to trick devices into initiating transactions.
How do you secure your Aadhaar biometric information?
- The UIDAI is proposing an amendment to the Aadhaar (Sharing of Information) Regulations, 2016, which will require entities in possession of an Aadhaar number to not share details unless the Aadhaar numbers have been redacted or blacked out through appropriate means, both in print and electronic form. The UIDAI has also implemented a new two-factor authentication mechanism that uses a machine-learning-based security system, combining finger minutiae and finger image capture to check the ‘liveness’ of a fingerprint.
- Additionally, users are also advised to ensure that they lock their Aadhaar information by visiting the UIDAI website or using the mobile app. This will ensure that their biometric information, even if compromised, cannot be used to initiate financial transactions.
- It can be unlocked when the need for biometric authentication arises, such as for property registration and passport renewals, after which it can again be locked.
What can be done in case of a financial scam using Aadhaar?
- If users have not already locked their Aadhaar biometric information, they should do so immediately in case of any suspicious activity in their bank accounts.
- Users are also advised to inform their banks and the concerned authorities as soon as possible. Timely reporting can ensure that any money transferred using fraudulent means is returned to the victim.
- The RBI in a circular has stated that a customer’s entitlement to zero liability arises where the unauthorised transaction occurs, and the customer notifies the bank within three working days of receiving a communication from the bank regarding such unauthorised transaction.
What is a transformer, the machine learning model that powers ChatGPT?
(GS Paper 3, Science and Technology)
Why in News?
- Machine learning (ML), a subfield of artificial intelligence, teaches computers to solve tasks based on structured data, language, audio, or images, by providing examples of inputs and the desired outputs.
- This is different from traditional computer programming, where programmers write a sequence of specific instructions. Here, the ML model learns to generate desirable outputs by adjusting its many knobs called parameters.
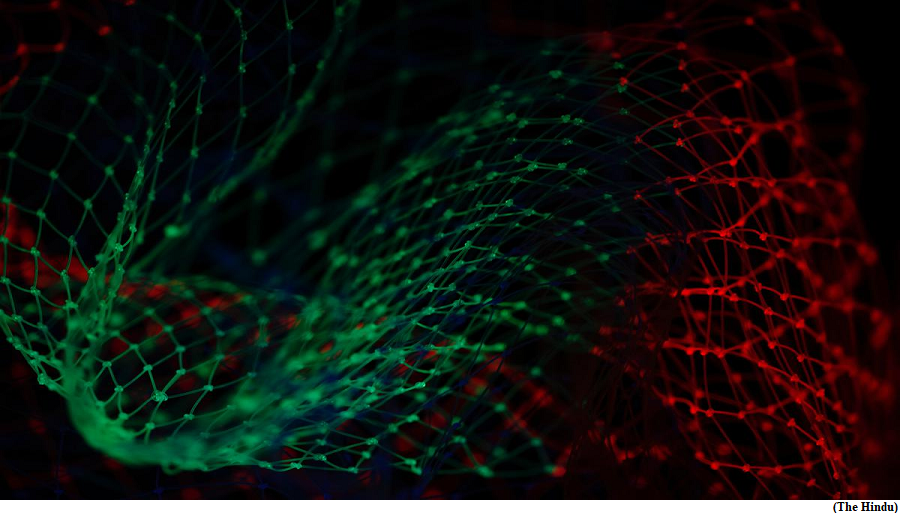
Deep neural networks:
- In the first part of the 2010s, deep neural networks (DNNs) took over ML by storm, replacing the classic pipeline of hand-crafted features and simple classifiers. DNNs ingest a complete document or image and generate a final output, without the need to specify a particular way of extracting features.
- While these deep and large models have existed in the past, their large size hindered their use. The resurgence of DNNs in the 2010s is attributed to the availability of large-scale data and fast parallel computing chips called graphics processing units.
- Furthermore, the models used for text or images were still different — recurrent neural networks were popular in language understanding while convolutional neural networks (CNNs) were popular in computer vision, that is, a machine understanding of the visual world.
Origin of transformers:
- In a pioneering paper entitled ‘Attention Is All You Need’ that appeared in 2017, a team at Google proposed transformers, a DNN architecture that has today gained popularity across all modalities (image, audio, and language).
- The original paper proposed transformers for the task of translating a sentence from one language to another, similar to what Google Translate does when converting a sentence from, say, English to Hindi.
How Transformer work?
- A transformer is a two-part neural network. The first part is an ‘encoder’ that ingests the input sentence in the source language (English) and the second part is a ‘decoder’ that generates the translated sentence in the target language (Hindi).
- The encoder converts each word in the source sentence to an abstract numerical form that captures the meaning of the word within the context of the sentence, and stores it in a memory bank.
- Both these processes use a mechanism called ‘attention’, hence the name of the paper. A key improvement over previous methods is the ability of a transformer to translate long sentences or paragraphs correctly. The adoption of transformers subsequently exploded.
- The capital ‘T’ in ChatGPT, for example, stands for ‘transformer’.
- Transformers have also become popular in computer vision as they simply cut an image into small square patches and line them up, just like words in a sentence. By doing so, and after training on large amounts of data, a transformer can provide better results than CNNs.
- Today, transformer models constitute the best approach for image classification, object detection and segmentation, action recognition, and a host of other tasks.
What is ‘attention’?
- Attention in ML allows a model to learn how much importance should be given to different inputs.
- In the translation example, attention allows the model to select or weigh words from the memory bank when deciding which word to generate next. While describing an image, attention allows models to look at the relevant parts of the image when generating the next word.
- A fascinating aspect of attention-based models is their ability for self-discovery, by parsing a lot of data.
- Transformers are attention models on steroids. They feature several attention layers both within the encoder, to provide meaningful context across the input sentence or image, and from the decoder to the encoder when generating a translated sentence or describing an image.
Applications of transformers:
- Since 2022, transformer models have become larger and train on more data than before. When these colossuses train on written text, they are called large language models (LLMs). ChatGPT uses hundreds of billions of parameters whereas GPT-4 uses hundreds of trillions.
- While these models are trained on simple tasks, such as filling in the blanks or predicting the next word, they are very good at answering questions, creating stories, summarising documents, writing code, and even solving mathematical word problems in steps.
Concerns:
- The scientific community is yet to figure out how to evaluate these models rigorously. There are also instances of “hallucination”, whereby models make confident but wrong claims.
- There is need to urgently address societal concerns, such as data privacy and attribution to creative work, that arise as a result of their use.